Most data lakes have emerged from incremental growth and experimentation. The idea of designing a data lake is something that few people have ever considered. The right approach to creating a data lake is to take the same approach as this white paper: follow the data. Or, perhaps more aptly, follow your data.
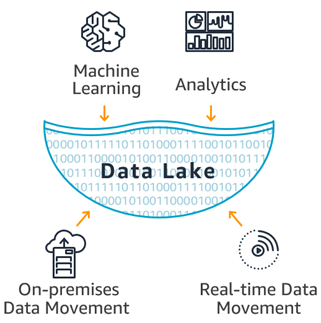
The path toward a data lake may differ based on where you start. Is your business entirely focused on big data? Or is big data just starting to come into view? Are you already a company with a data-driven, analytics culture? Or are you building muscle with respect to exploiting data?
The following stages represent the path that many companies take as they start to implement a data lake.
Stage 1:
Handling data at scale. The first stage involves getting the plumbing in place and learning to acquire and transform data at scale. In this stage, the analytics may be quite simple, but much is learned about making Hadoop work the way you desire.
Stage 2:
Building transformation and analytics muscle. The second stage involves improving the ability to transform and analyze data. In this stage, companies find the tools that are most appropriate to their skill set and start acquiring more data and building applications. Capabilities from the enterprise data warehouse and the data lake are used together.
Stage 3:
Broad operational impact. The third stage involves getting data and analytics into the hands of as many people as possible. It is in this stage that the data lake and the enterprise data warehouse start to work in unison, each playing its role. One example of the need for this combination is the fact that almost every big data company that started with a data lake eventually added an enterprise data warehouse to operationalize its data. Similarly, companies with enterprise data warehouses are not abandoning them in favor of Hadoop.
Stage 4:
Enterprise capabilities. In this highest stage of the data lake, enterprise capabilities are added to the data lake. Few companies have reached this level of maturity, but many will as the use of big data grows, requiring governance, compliance, security, and auditing.
Conclusion
By following the data, we have shown that the emergence of the data lake comes from the need to manage and exploit new forms of data. The shape of your data lake is determined by what you need to do but cannot with your current data processing architecture. The right data lake can only be created through experimentation. Together, the data lake and the enterprise data warehouse provide a synergy of capabilities that delivers accelerating returns. Allowing people to do more with data faster and driving business results: That’s the ultimate payback from investing in a data lake to complement your enterprise data warehouse. Logical data warehouses deliver the ability to know more about your business and gain more value from all your data.
Credits: CITO Research